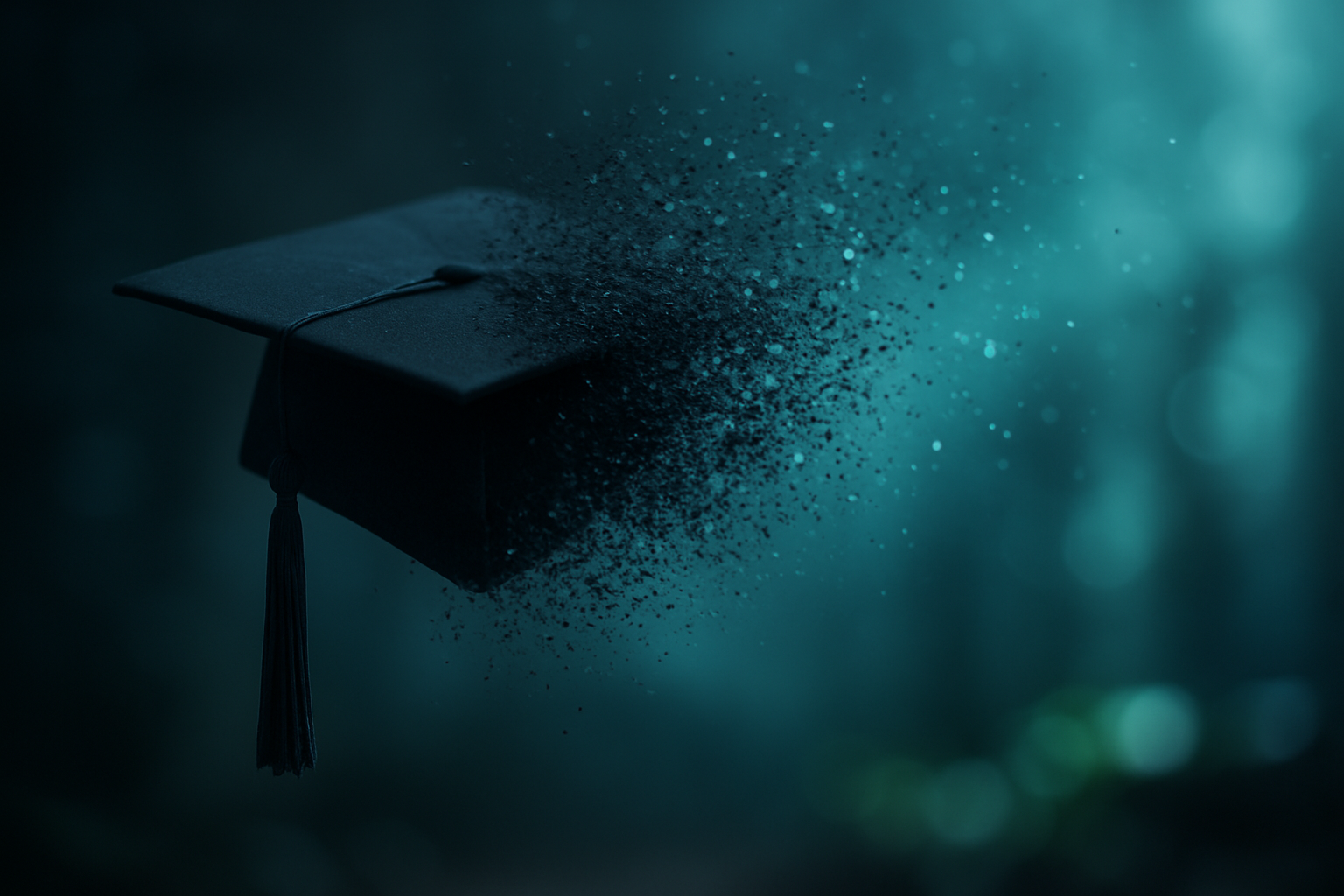
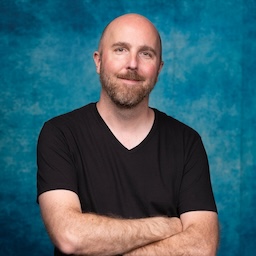
Does the modern university end at AI?
AI Trends in Higher Education,” hosted by Emerald Publishing and the Higher Education Teaching and Learning Association (HETL), March 26, 2025
What If we’re witnessing the end of the modern university?
The webinar AI Trends in Higher Education brought together educators, researchers, and AI specialists from across the globe to discuss how artificial intelligence is reshaping the foundations of higher education. With over 500 attendees from institutions around the world, the event spotlighted urgent questions that many universities have barely begun to ask, let alone answer.
I joined Christine O’Dea, Mariann Hardey, and Ahmed Hassan Afridi in a session moderated by Abhilasha Singh. While each of us approached AI from different angles (e.g., equity, governance, research, policy) the shared conclusion was clear: AI is a force reconfiguring the logic of higher education itself. And I sense we left the webinar with far more questions about the future of higher education than when we entered.
Beyond the buzz: What’s changing?
While much of the discussion on AI focuses on tools—chatbots, grading assistants, recommendation engines—the deeper shift is structural. I raised three trends that are gaining momentum but haven’t yet entered the mainstream conversation:
- AI-led institutional governance:
We’re starting to see AI systems support and inform strategic decisions at the institutional level (budgets, policy, even faculty allocation). That begs the question: who’s really in charge? - Integration with other disruptive technologies:
AI has distracted us from other paradigm-shifting technologies. Combine AI with blockchain, and suddenly we’re talking about self-updating credentials and decentralized learning records that follow students across borders and careers. - The creeping commercialization of learning:
AI platforms are increasingly controlled by corporations whose priorities don’t align with academic freedom or equity. If we’re not careful, we may end up outsourcing curriculum design, faculty performance evaluations, and student support to algorithms designed for scale—not care.
Who benefits …and who’s left behind?
We often hear that AI will “democratize education.” It’s a comforting idea: that machine learning can help level the playing field, offering personalized learning at scale, freeing up faculty time, and making education more accessible. But democratization implies equal power—and AI systems don’t operate in neutral terrain. Somebody always owns the tool we use. Thus, it is important to keep in mind that democratization does not always equal democracy.
AIs are built by humans, trained on historical data, and deployed within institutions shaped by long-standing hierarchies. Which means they tend to replicate the assumptions (and the blind spots) of the systems they emerge from.
During the panel, I emphasized the risk of algorithmic conformity: the tendency of AI-driven tools to reward students who fit existing molds. Learners who think differently, whose cultural or linguistic backgrounds diverge from the majority, or who express understanding in nontraditional ways are often penalized, not because they lack ability, but because they fall outside the model’s training data.
And the stakes are higher than we admit. If predictive analytics flag certain students as “at risk,” does that become a self-fulfilling prophecy? If AI-generated feedback favors certain writing styles or problem-solving approaches, whose knowledge is validated—and whose is erased?
The tools themselves may not be biased intentionally by design, but the data they’re trained on often is. Most large models draw disproportionately from English-language, Western, and male-authored sources. That’s not a technical glitch. It’s a systemic design flaw. And unless institutions invest in equity audits, transparent model evaluation, and diverse stakeholder involvement—including students—we risk reproducing existing inequities, but this time at scale.
It’s not enough to ask whether a system “works.” We have to ask: For whom? In what contexts? And at what cost?
If we want AI to support inclusion, it must be intentionally designed, tested, and governed for it. Otherwise, the promise of “personalized learning” becomes a veneer over systems that quietly sideline the very learners who could benefit most.
Reimagining the role of the university
If we were to design higher education from scratch in an AI-driven world, would it look anything like what we have now?
It’s unlikely. The industrial-era blueprint that still shapes most universities (structured degrees, rigid semesters, siloed disciplines, and assessment models built around scarcity and standardization) was not built for a world of continuous, distributed learning. Nor was it built for a world where intelligent systems can generate, translate, evaluate, and even co-create knowledge on demand.
In an AI-augmented future, the university might be:
- More decentralized with learning experiences happening across platforms, locations, and life stages. Institutions may no longer hold a monopoly on learning but instead function as hubs—certifying, curating, and connecting rather than solely delivering education.
- More personalized with students navigating dynamic learning pathways shaped by real-time data, feedback loops, and intelligent recommendation systems. The idea of the “average learner” may disappear entirely.
- Less bound to rigid degree structures, with micro-credentials, portfolios, and skills-based recognition gradually replacing or supplementing traditional qualifications. Lifelong learning could move from rhetoric to infrastructure.
- More integrated with the world beyond academia, with universities partnering directly with communities, industries, and global networks to co-design learning experiences rooted in relevance and application—not just theory.
In this scenario, faculty roles also shift (though not quite into obsolescence). Rather than functioning solely as content experts, educators may become learning designers, cognitive coaches, and AI mediators, guiding students in how to interrogate, interpret, and apply machine-generated knowledge. Their value lies not in outpacing machines, but in helping humans make sense of the flood of information AI systems produce.
Just as importantly, institutions themselves may need to compete on academic quality as well as on their ethical positioning: how they govern the use of AI, protect learner data, center inclusion, and promote meaningful human agency in a machine-augmented world.
This doesn’t mean AI will replace education, or educators. But it will redefine what counts as learning, who gets to provide it, and how that learning is valued by institutions, by employers, and by learners themselves.
The university of the future may not be a place. It may be a network. A platform. A set of relationships and commitments. And the most resilient institutions will be those that recognize this shift not as a threat, but as an invitation to evolve.
Moving forward: A call for strategic imagination
The good news? We don’t need to be passive recipients of technological change. Education is not a tech industry waiting to be disrupted. It is a social institution, shaped by human values, cultural context, and collective choice. And that means we still have a critical window to shape AI’s role in higher education before it shapes us.
But doing so will require more than policy updates or tool adoption. It will demand strategic imagination: the ability to think beyond immediate efficiencies and short-term gains, and to design for the kind of future we actually want to inhabit.
That starts with some foundational shifts:
- Building institutional capacity to experiment wisely:
Too often, AI initiatives are driven top-down or outsourced entirely. Institutions need internal capacity (in IT departments and across academic and administrative units) to test, evaluate, and iterate with new tools. This includes spaces for low-risk experimentation, pilot programs, and cross-functional AI literacy development that moves beyond one-off workshops. - Developing policies rooted in human values, not machine compliance:
Governance frameworks must go further than outlining what is “allowed.” They need to address what is just, inclusive, and educationally sound. That means refocusing toward policies that center academic integrity, student agency, privacy protections, and transparent algorithmic decision-making, especially in areas such as admissions, assessment, and advising. - Fostering interdisciplinary collaboration as a core institutional competency:
AI doesn’t live in a single department. Nor does its impact. Bringing together educators, technologists, ethicists, students, and policymakers is a necessity. This means creating structures that reward collaborative inquiry across silos and treat ethical foresight as a key form of leadership.
Strategic imagination also requires a shift in narrative. Rather than asking whether AI will replace us (or how we can “keep up”) we need to ask: What kind of learning ecosystem do we want to build? Who is it for? And what do we refuse to optimize?
Because that’s the real danger; not that we’ll use AI, but that we’ll use it to optimize the wrong things: Speed over depth. Efficiency over equity. Prediction over possibility.
The path forward us to reclaim our agency in how we engage with AI, not about resisting technology or adapting it for the sake of adoption. Educational transformation will not come from the tools we adopt. It will come from the values we amplify.